成果速递:博士生冯家辉提出自适应共享单车流量预测方法
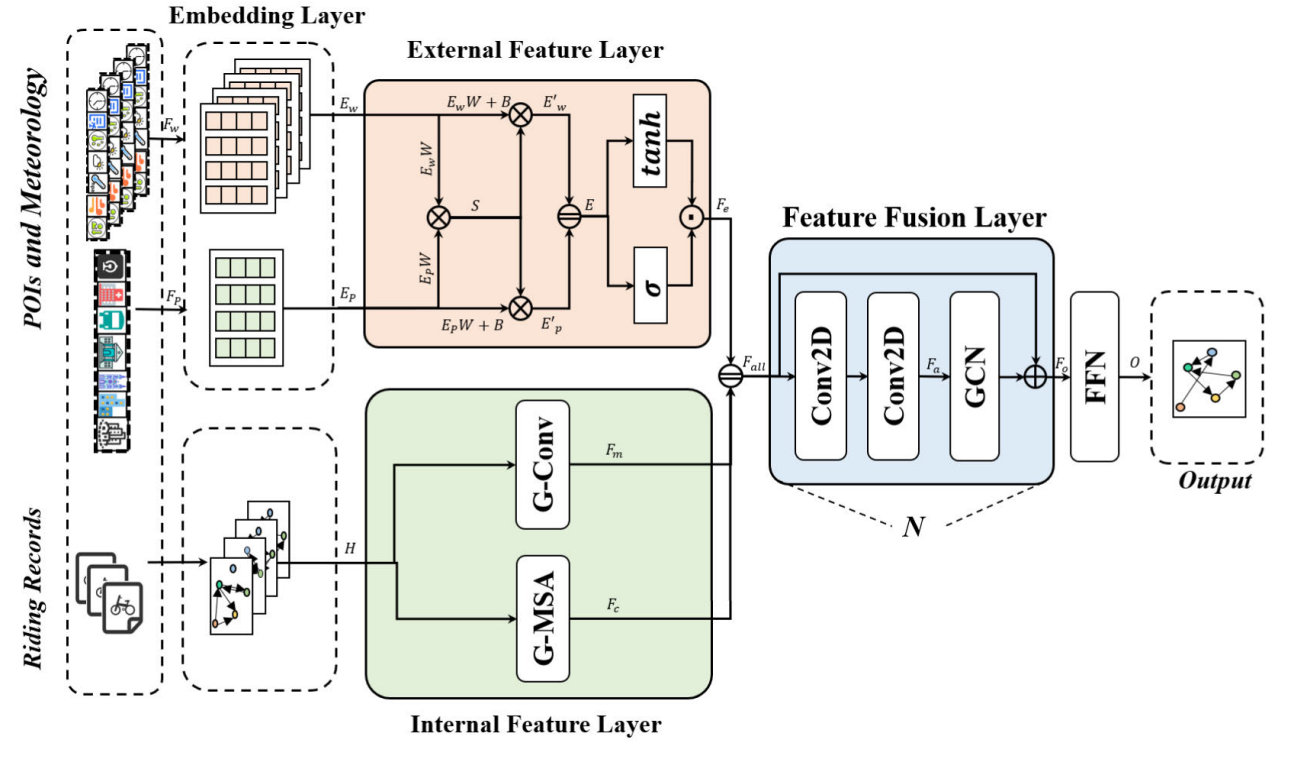
Title: An Adaptive Spatial-Temporal Method Capturing for Short-Term Bike-Sharing Prediction;
Authors: Jiahui Feng, Hefu Liu*;
Journal: IEEE Transactions on Intelligent Transportation Systems;
URL: https://doi.org/10.1109/TITS.2024.3406682;
Abstract:
Shared bike prediction has been a crucial task in shared bike management, and many deep learning algorithms have shown promise in improving the precision of bike flow predictions. But the shared bike riding records are highly sensitive to meteorological and built environment factors. Additionally, the number of active stations at different times of the day varies widely, with most stations active during the day and only a few active at night, which needs to focus on local and global bike-sharing system information. Existing methods rarely consider both meteorological and built environment features, and overlook the local and global changes of bike-sharing systems in different time intervals. To address these challenges, we propose an adaptive spatial-temporal network to predict the number of rented and returned bikes at the station level. This paper introduces a group multi-head self-attention mechanism (G-MSA) to divide all stations into small patches to capture the local and global spatial information. We also propose two types of adaptive adjacent matrices to reflect the dynamic connections between stations and the impact of external factors on shared bike flows. The proposed model is evaluated on four real-world shared bike datasets with varying time intervals, and the results outperform benchmark models. Moreover, ablation experiments also demonstrate the effectiveness of G-MSA and the varying importance of meteorological features and built environment features across BSSs in different cities.
Acknowledgement: This work was supported by the National Natural Science Foundation of China (NSFC) under Grant 72332007, Grant 72272139, Grant 71971202, and Grant 71921001.