成果速递:博士生吴俊涛提出两阶段多渠道零售销量预测方法
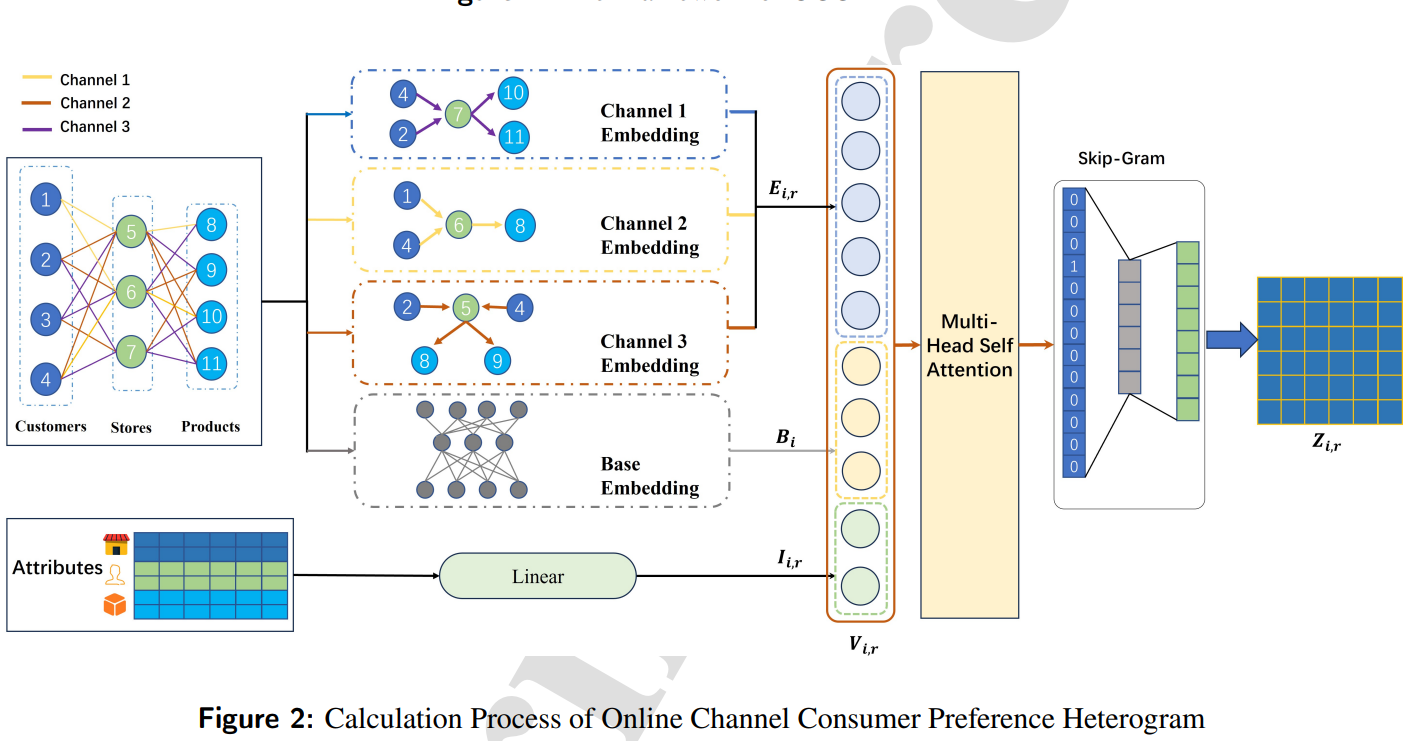
Title: Unveiling consumer preferences: A two-stage deep learning approach to enhance accuracy in multi-channel retail sales forecasting;
Authors: Juntao Wu, Hefu Liu, Xiaoyu Yao, Liangqing Zhang*;
Journal: Expert Systems with Applications;
URL: https://doi.org/10.1016/j.eswa.2024.125066;
Abstract:
In the dynamic and turbulent business environment, sales forecasting for multi-channel retailers has become increasingly intricate, particularly with the shift from traditional brick-and-mortar channels to a diverse range of distribution channels. This transition not only complicates forecasting challenges but also highlights the crucial role of utilizing online traceable consumer purchase data to discern consumer preferences for stores and products and enhance sales forecasting accuracy. This paper proposes a two-stage deep learning approach based on Online Channel Consumer Preference Heterogram and Multi-Head Attention mechanism (OCCPH-MHA). In the first stage, the model identifies potential consumer group preferences based on individual purchasing behavior. In the second stage, it seamlessly integrates this identified feature with time-series demand data using a global-local attention mechanism, thereby facilitating multi-step forecasting. This study’s robust validation involves testing the model on the dataset from a multi-channel retail restaurant company, showcasing its prowess in significantly improving the precision of sales forecasting. This not only substantiates the model’s effectiveness but also underscores the importance of consumer group preferences, as it contributes to a comprehensive framework. This framework, focused on tracking the preferences of potential consumer groups, emerges as a valuable tool that collectively refines and optimizes the sales forecasting process for both industry practitioners and researchers.
Acknowledgement: This work is supported by the Grants from the National Natural Science Foundation of China (grant number 72332007) and the China Postdoctoral Science Foundation (grant number 2024M753853).