成果速递:博士生冯家辉提出基于时空聚合图神经网络的共享单车需求预测方法
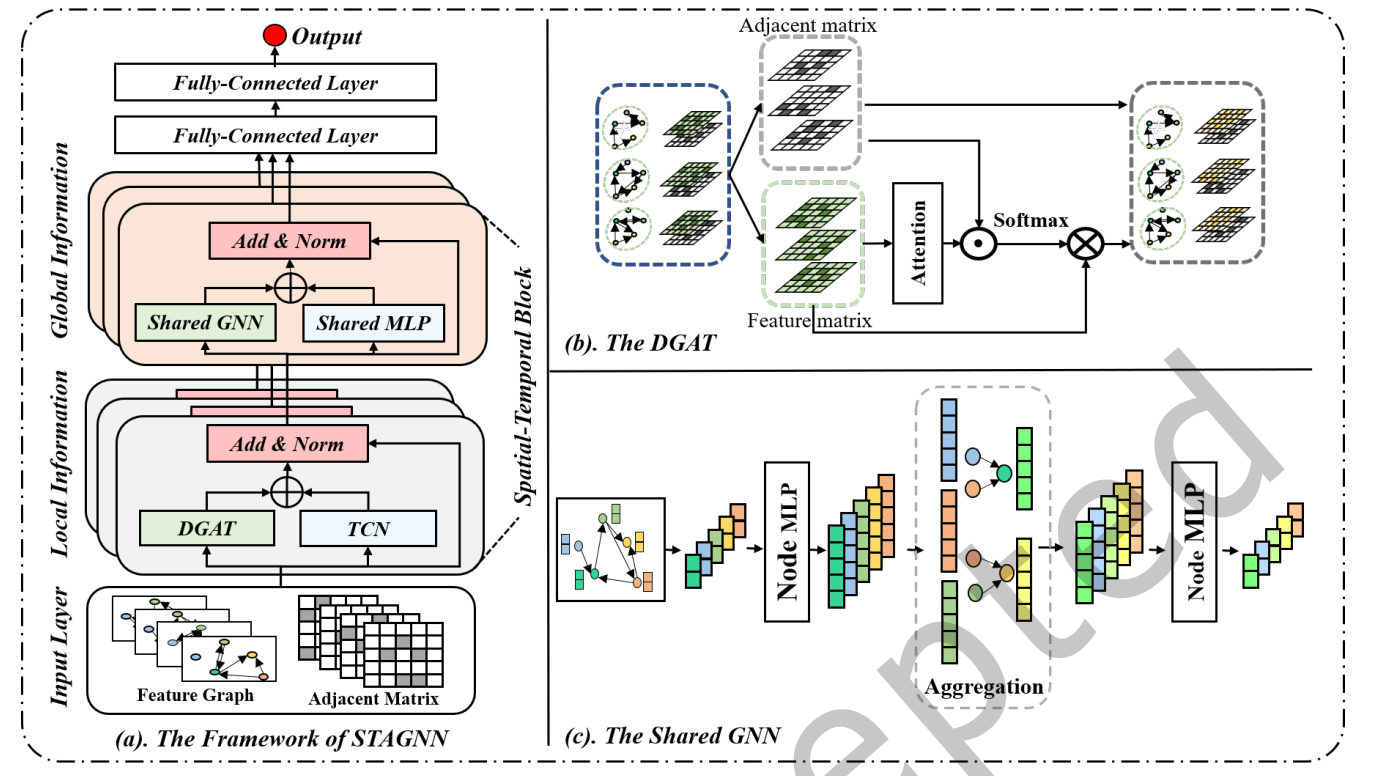
Title: A Spatial-Temporal Aggregated Graph Neural Network for Docked Bike-sharing Demand Forecasting;
Authors: Jiahui Feng, Hefu Liu, Jingmei Zhou*, Yang Zhou;
Journal: ACM Transactions on Knowledge Discovery from Data;
URL: https://doi.org/10.1145/3690388;
Abstract:
Predicting the number of rented and returned bikes at each station is crucial for operators to proactively manage shared bike relocation. Although existing research has proposed spatial-temporal prediction models that significantly advance traffic prediction, these models often neglect the unique characteristics of shared bike systems (BSS). Spatially, the entire Bike Sharing System (BSS) experiences peak activity during morning and evening rush hours, whereas, during other periods, activity is localized to local stations, with some recording no rides, highlighting the need to distinguish between global and local spatial information across different times. Temporally, the historical riding records for each station exhibit non-stationary patterns, necessitating the analysis of both global trends and local fluctuations. Existing Graph Neural Network (GNN) approaches to predicting shared bike demand primarily capture static spatial-temporal data and fail to account for the dynamic nature of bike flows. Moreover, these studies focus on global spatial-temporal information without considering local nuances, making it challenging to capture spatiotemporal dynamics in fluctuating BSS. To address these challenges, we introduce the Spatial-Temporal Aggregated Graph Neural Network (STAGNN). Our model first constructs a dynamic adjacent matrix to describe the evolving connections between stations, followed by local and global information layers to capture spatial-temporal information from large-scale shared bike networks accurately. Our methodology has been validated through experiments on four real-world datasets, comparing it against benchmark models to demonstrate superior prediction accuracy. Additionally, we conduct extended experiments on four datasets during the morning and evening rush hours, and the results also affirm the efficacy of the STAGNN in enhancing prediction performance.